Infer Lead Scoring: A Comprehensive Analysis
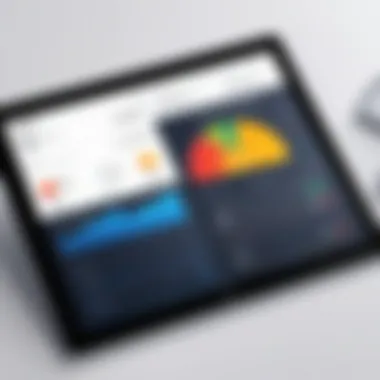
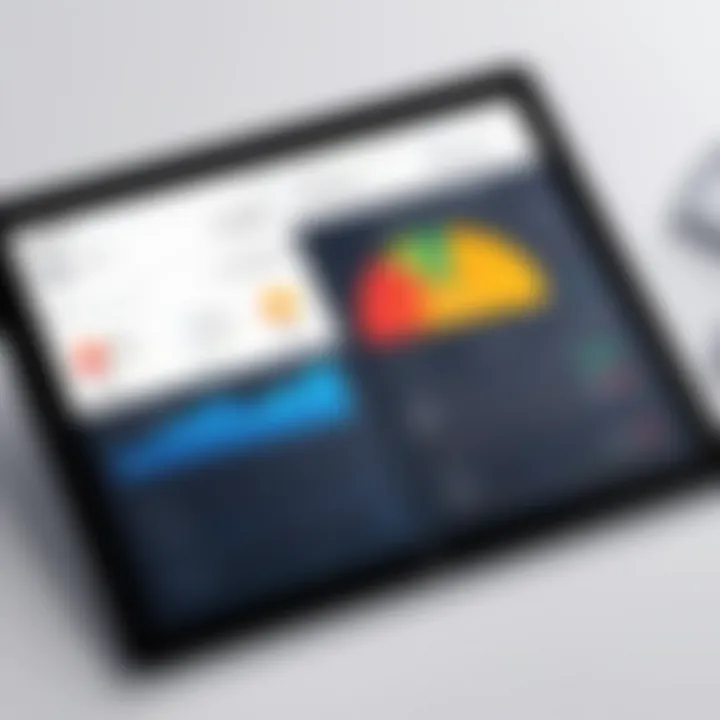
Intro
Infer lead scoring is emerging as a critical methodology within B2B marketing and sales. At its core, it aims to quantify the potential of leads, thereby allowing businesses to prioritize their efforts and maximize conversion rates. This process is not just about numbers; it integrates a mix of behavioral and demographic data to craft a more nuanced view of leads.
The increasing complexity of buyer behavior necessitates a robust system that accurately assesses lead quality. Businesses today require not just tools, but also strategic insights to navigate this landscape. Thus, this article will provide an in-depth analysis, emphasizing the significance of infer lead scoring in the decision-making process.
In the following sections, we will dissect various components of infer lead scoring, including its foundational features, pricing structures, and practical implementation strategies. By providing concrete examples and addressing common challenges, this discussion will serve as a comprehensive resource for decision-makers and stakeholders seeking to enhance their lead management practices.
Understanding Infer Lead Scoring
Understanding infer lead scoring is essential for organizations navigating the complexities of B2B marketing and sales. This concept serves as a crucial framework for evaluating potential leads and prioritizing them based on their likelihood to convert into customers. By implementing an effective lead scoring model, businesses can streamline their sales processes, improve resource allocation, and ultimately enhance overall sales performance.
Definition and Relevance
Infer lead scoring refers to a systematic approach that quantifies the potential value of leads, utilizing various data points to predict their probability of conversion. This model helps sales teams determine which leads to pursue with urgency and which to nurture over time. The relevance of this method lies in its ability to provide insights that are data-driven rather than intuition-based. It enhances decision-making processes, allowing businesses to focus their resources more effectively.
Key Elements of Infer Lead Scoring:
- Data-Driven Insights: Leverage customer data to establish clear criteria for scoring leads.
- Customization: Adapt the scoring model to fit specific business goals and market conditions.
- Efficiency: Improve workflow by enabling sales teams to concentrate on high-potential leads.
Historical Context
The concept of lead scoring is not new. It emerged in response to the increasing complexity of marketing and sales strategies fueled by technological advancements in the digital era. Historically, companies relied on demographic information and gut feelings to qualify leads. However, as markets evolved, so did the need for more analytical approaches.
With the advent of Customer Relationship Management (CRM) software and marketing automation tools, businesses began to integrate data from multiple sources, enabling more sophisticated scoring systems. This evolution marked a shift towards more predictive techniques that could assess leads based on behaviors and interactions.
In the current landscape, the sophistication of lead scoring continues to advance with the integration of artificial intelligence and machine learning. These technologies push the boundaries of data utilization, helping organizations not only to score leads but to refine their strategies further based on changing market dynamics. Understanding where infer lead scoring develops from provides crucial insights into its importance in modern business practices.
In summary, the understanding of infer lead scoring enhances a business's ability to convert leads into customers effectively. By acknowledging its definition, relevance, and historical significance, stakeholders can appreciate the transformative role this model plays in shaping successful sales strategies.
The Need for Lead Scoring in B2B
The context of B2B marketing continuously evolves, driven by advancements in technology and shifts in consumer behavior. Sales teams encounter growing sets of data that, if utilized correctly, can contribute greatly to efficient sales processes. Lead scoring becomes a fundamental strategy in this landscape. It enhances the ability to prioritize leads based on their likelihood to convert, leading to more focused resource allocation and ultimately improved sales outcomes.
Challenges in Lead Management
Lead management presents various challenges for businesses. One notable issue is the abundance of leads generated through diverse channels. Not all leads are equally valuable; some may show high engagement but lack the budget or authority to make decisions. Furthermore, sales professionals often face the problem of distinguishing between warm leads and those that may only be interested at a surface level. Other challenges include:
- Lack of alignment between marketing and sales teams, which can lead to miscommunication about lead quality and expectations.
- Data overload, resulting in analysis paralysis. The rich array of data can overwhelm teams, diluting focus on leads that actually convert.
- Inconsistent lead qualification criteria, which can make it hard to standardize efforts across the team.
These challenges underscore the necessity for a robust lead scoring system. With such a system, companies can address these obstacles effectively.
The Role of Lead Scoring
Lead scoring acts as a tool to assign value to each lead based on specific criteria. It allows organizations to filter leads and focus on those likely to convert into sales. Using a lead scoring model, businesses can evaluate leads based on their behavior and demographics. This ensures that sales teams concentrate efforts on leads with the highest potential.
Key benefits of implementing lead scoring include:
- Efficient resource allocation: Sales teams can devote their time to high-potential leads, resulting in increased conversion rates.
- Improved marketing efforts: Insights gleaned from lead scoring can help marketing teams tailor their strategies, effectively reaching and engaging potential customers.
- Enhanced customer experience: Personalization and relevant communication become possible, elevating the relationship between the business and leads.
By addressing the inherent challenges within lead management through effective scoring, businesses can significantly improve their sales processes and outcomes. The systematic weight assigned to various lead attributes makes for a refined approach to nurturing leads toward conversion.
Data Sources for Lead Scoring
In the realm of lead scoring, understanding and leveraging the right data sources is crucial. Data sources provide the foundation upon which lead scoring models are built. They dictate how accurately a business can predict the likelihood of a lead converting into a customer. Utilizing both internal and external data resources can enhance the lead scoring process significantly, leading to improved decision-making and ultimately, higher conversion rates.
The importance of data sources in lead scoring cannot be understated. They enable organizations to profile potential leads effectively, categorize them based on various metrics, and tailor marketing efforts accordingly. Inaccurate or incomplete data can lead to misguided strategies. Hence, careful selection and continuous refinement of these sources must be prioritized.
Internal Data Utilization
Internal data is often the most accessible and relevant data source a company can utilize. It involves information collected from previous engagements, sales history, customer interactions, and even website behavior. Such data provides insights into existing customers, including their purchasing patterns and preferences.
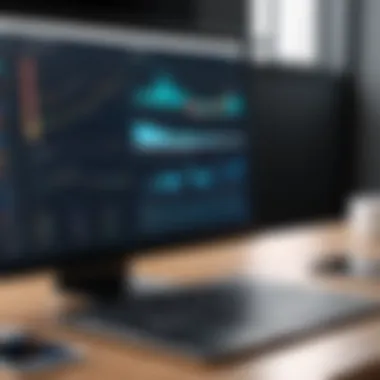
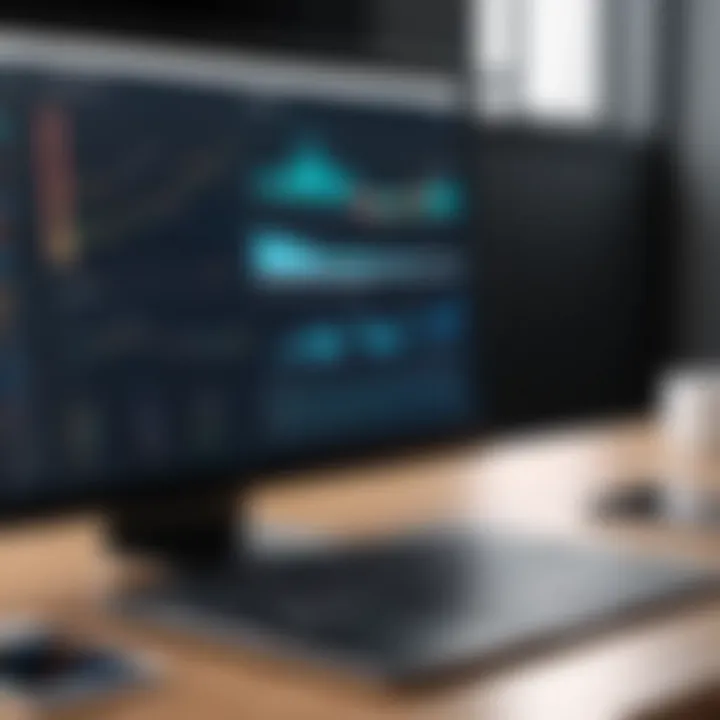
Key elements of internal data utilization include:
- CRM Systems: Many businesses rely on Customer Relationship Management tools to track interactions and sales. Integrating lead scoring into these systems can yield insights into lead responsiveness.
- Historical Sales Data: Analyzing past sales can reveal characteristics of leads that typically convert. This analysis can inform weighting and scoring.
- Employee Feedback: Input from sales teams can provide qualitative insights regarding lead quality. Their observations can often highlight which factors are most predictive of a successful sale.
Effective internal data utilization streamlines the lead scoring process. However, it is not without limitations. Internal data can sometimes be biased or incomplete. Hence, it should be complemented by external resources to ensure a more rounded perspective.
External Data Resources
On the other hand, external data sources bring in additional layers of information and context to lead scoring. External data can provide a broader understanding of the market landscape, industry trends, and outside behaviors that may influence lead interaction.
Considerations for utilizing external data include:
- Third-party Data Providers: Services such as ZoomInfo or LinkedIn Sales Navigator offer enriched data profiles of potential leads. This can help in identifying prospect quality.
- Social Media Insights: Social platforms can provide data about user engagement and interests. This information can be vital in understanding lead fit.
- Market Research Reports: These reports can help companies understand industry trends, which can in turn impact lead behavior and scoring methodologies.
Relying on external data sources enhances the reliability of lead scores by introducing fresh perspectives. Still, companies must ensure compliance with data privacy regulations when utilizing these resources. Data validation processes are also crucial to avoid relying on outdated or incorrect data, which could mislead scoring efforts.
In summary, integrating both internal and external data sources into lead scoring methodologies promotes a comprehensive and effective approach to managing leads. By continually updating and refining these data sources, businesses can maintain competitive advantage in lead generation and conversion strategies.
"Data is the new oil, it is valuable but if unrefined it cannot really be used."
- Clive Humby
Employing a mix of both internal and external data lays the groundwork for a robust lead scoring system that adapts to changing market demands.
Establishing a Lead Scoring Model
In todayβs competitive B2B landscape, establishing an effective lead scoring model is crucial for maximizing sales efficiency and driving revenue growth. This model helps organizations identify which leads are most likely to convert based on various data points and behaviors. A properly designed lead scoring model not only streamlines sales efforts but also aligns marketing strategies, ensuring that resources are allocated efficiently.
Defining Key Metrics
Defining key metrics is the foundation of a successful lead scoring system. Key metrics often include demographic information, engagement levels, and buying signals. Here are some common metrics to consider:
- Demographics: Understanding lead profiles based on criteria like industry, company size, and location provides valuable context.
- Engagement Levels: Tracking how leads interact with your content (emails opened, website visits, etc.) can reveal their level of interest.
- Buying Signals: Identifying signals such as requests for demos or price quotes can indicate readiness to purchase.
By establishing these metrics, a company can tailor its marketing strategies to align with lead behavior effectively. This effort will pave the way for a more refined approach to scoring leads.
Weighting System Development
Once key metrics are established, the next step is to develop a weighting system. This system assigns different values or weights to each metric based on its relevance to the buying process. Not all metrics carry the same importance, thus a careful assessment is essential.
- Determine Importance: Analyze historical data to see which metrics correlate strongly with successful conversions.
- Assign Weights: Assign numerical values to metrics, with higher values indicating greater importance. For instance, a download of a whitepaper might carry more weight than a simple email open.
- Create a Scoring Formula: Develop a formula that combines these weighted metrics to produce an overall score for each lead. This formula is where the lead scoring model materializes into a practical tool for sales teams.
To be effective, ensure that the scoring model evolves over time. Regularly revisit the assigned weights and metrics to adapt to shifting market trends and buyer behavior.
These steps in establishing a lead scoring model are vital to ensuring the model is robust and applicable. A well-structured lead scoring model not only assists in identifying high-potential leads but also enhances overall sales strategy effectiveness.
Implementing the Scoring System
Implementing a lead scoring system is crucial for optimizing the sales process. This system not only assists in identifying which leads are the most promising but also provides a structured approach to managing potential customer relationships. The effective implementation of lead scoring hinges on a few specific elementsβintegration with Customer Relationship Management (CRM) systems and training sales teams.
Integration with CRM Systems
Integrating a lead scoring system with existing CRM platforms is necessary for streamlining the data flow. A well-implemented integration ensures that leads are scored in real-time, allowing sales teams to focus on high-priority opportunities. Here are some key benefits of such integration:
- Seamless Data Synchronization: Automated syncing of lead data reduces manual entry and minimizes the risk of errors.
- Enhanced Lead Visibility: Sales representatives can easily see a leadβs score alongside historical interaction data, facilitating informed decision-making.
- Improved Follow-up: Proper integration allows for automated follow-up processes, which can enhance engagement with highscores leads.
However, there are considerations to keep in mind:
- Compatibility: Ensuring that the lead scoring system is compatible with the current CRM is essential to avoid integration issues.
- Training: Staff must be educated on how to utilize new features provided by the integration to maximize its potentials.
Training Sales Teams
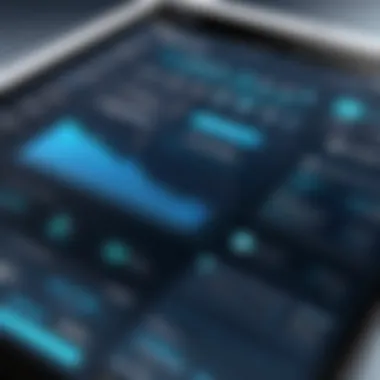
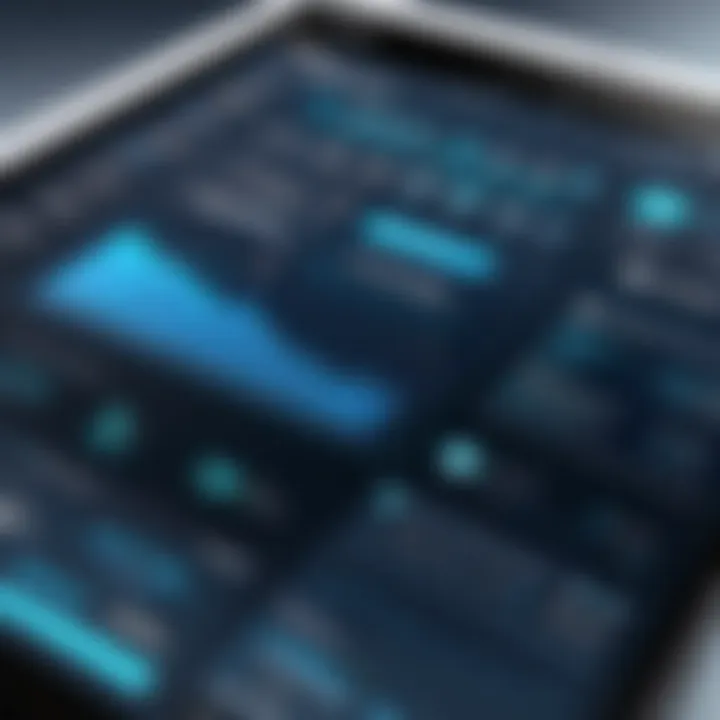
Even with advanced systems in place, the effectiveness of lead scoring largely relies on how well sales teams understand and utilize it. Training is therefore critical and should encompass several important facets:
- Understanding Lead Scores: Sales personnel must grasp the implications of various score levels. Not all leads are equal, and knowing which leads to prioritize is essential for effective sales strategies.
- Leveraging CRM Tools: Training should focus on how to utilize CRM features introduced by integration. This training can cover how to locate leads based on their scores and how to track interactions efficiently.
- Consistent Feedback Loop: Sales teams should be encouraged to provide feedback based on their experiences with scored leads. This data can help refine the scoring model over time, enhancing accuracy and efficiency.
By investing in training, organizations not only empower their sales teams but also ensure that the lead scoring model is leveraged effectively.
In summary, implementing a lead scoring system is about harmonizing technology with human expertise. Ensuring integration with CRM systems and providing adequate training for sales teams cultivates an environment of informed decision-making and strategic resource allocation.
Monitoring and Refining Lead Scores
Monitoring and refining lead scores is a crucial step in optimizing the lead scoring process. It ensures that the scoring system remains relevant and effective over time. Given the dynamic nature of markets, consumer preferences, and business environments, regular assessment and adaptation of lead scores are vital.
The process begins with establishing data analysis techniques. Effective monitoring requires a systematic approach to evaluating the data that influences lead scoring. Businesses often rely on metrics such as customer engagement, lead behavior, and conversion likelihood. Metrics should not remain static; they need to reflect the changing landscape.
Data Analysis Techniques
Data analysis techniques play a crucial role in the monitoring phase of lead scoring. Advanced analytics can help teams understand which scores correlate with successful conversions. This may include:
- Descriptive analytics: This is useful for summarizing historical data. It can show how past leads have performed in terms of conversion rates and what factors influenced those outcomes.
- Predictive analytics: Using machine learning algorithms, companies can predict future lead behavior based on historical data. This allows businesses to proactively adapt their lead scoring.
- Segmentation analysis: Grouping leads based on their characteristics can reveal trends and patterns in different segments. This helps in giving tailored scoring models relevant to each segmentβs unique needs.
Regularly applying these techniques allows businesses to identify gaps in their scoring models and make informed adjustments. An analytical review should occur at regular intervals, ensuring that the lead scoring system does not become outdated.
Adapting to Market Changes
Adapting to market changes is another fundamental aspect of maintaining an effective lead scoring system. Market trends, technological advancements, and shifts in consumer behavior can all affect lead quality. Businesses must remain agile to capture these changes effectively. Continuous feedback from sales and marketing teams can aid in recognizing these shifts. Sellers often have firsthand knowledge of changing lead behaviors, which can inform better scoring adjustments.
To ensure a responsive lead scoring system, consider the following:
- Regular feedback cycles: Implement structured feedback loops with marketing and sales teams. This ensures everyone is on the same page regarding lead quality.
- Market research: Stay updated on industry trends that may influence lead effectiveness. This includes competitor actions and emerging technologies.
- Iterative testing: Constant testing and iteration of scoring criteria can help identify what works best in the current market environment. This is an ongoing process that keeps scoring models aligned with actual market conditions.
Effective monitoring and refining of lead scores does not just improve conversion rates but can also significantly enhance resource allocation across departments. Timely and relevant adjustments create a scoring model that optimally positions the sales team to succeed. Thus, the impact of monitoring techniques and adaptation strategies on lead scoring cannot be overstated.
An adaptive lead scoring model is essential for staying competitive in the fast-paced B2B market. Regular monitoring helps anticipate shifts and prepare strategies to respond.
The Impact of Infer Lead Scoring
Leveraging infer lead scoring transforms how businesses approach potential customers. This concept is indispensable in B2B marketing. The primary aim is to enhance conversion rates and optimize resource allocation. Infer lead scoring enables a company to identify high-value leads. Rather than treating all leads equally, businesses can focus efforts on those most likely to convert.
Effective lead scoring directly correlates with measurable increases in conversion rates. When a business understands which leads demonstrate engagement, the sales process becomes more efficient. It allows for a more tailored approach, connecting prospects with targeted messaging. Not only does this improve customer experience, but it also maximizes the potential return on investment.
Additionally, it assists teams in making informed decisions regarding where to allocate resources. This means ensuring that marketing and sales teams are not spread too thin. Instead of attempting to nurture every contact, focusing on leads that exhibit a strong likelihood to convert ensures better use of budget and time.
"Effective lead scoring is a strategic move in marketing, leading to significant improvements in both conversion rates and resource management."
Conversion Rate Improvement
The correlation between infer lead scoring and conversion rate improvement is clear. By understanding the underlying behavior of leads, businesses can prioritize their outreach. This results in more meaningful interactions. Scoring helps identify which leads are not just interested but are ready to purchase.
Implementing this strategy can help increase conversion rates by:
- Focusing Efforts: Concentrating on leads with a higher score means engaging with prospects who are more likely to respond favorably.
- Personalized Communication: High-scoring leads often warrant tailored messages, addressing their specific needs and challenges.
- Timely Follow-Ups: Recognizing when leads are more engaged allows companies to act quickly, improving their chances of conversion.
Careful analysis of scored leads reveals patterns in behaviors and preferences. Understanding these trends enables marketing teams to craft more effective campaigns, potentially pushing conversion rates even higher.
Resource Allocation Efficiency
Efficient resource allocation is critical in today's competitive market. Infer lead scoring plays a vital role by streamlining how resources are distributed across the lead funnel. It allows businesses to avoid wasteful spending on low-priority leads.
With lead scoring, organizations can:
- Enhance Team Productivity: Sales teams can dedicate time to prospects that carry the highest scores, leading to a more focused approach.
- Reduce Costs: By honing in on high-potential leads, businesses can maximize their marketing budgets, reducing overall costs.
- Optimize Marketing Strategies: Evaluating lead scoring data guides marketing efforts, enabling precise strategy adjustments.
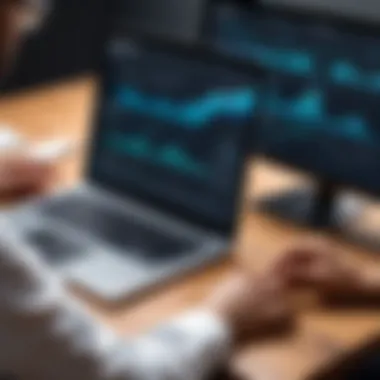
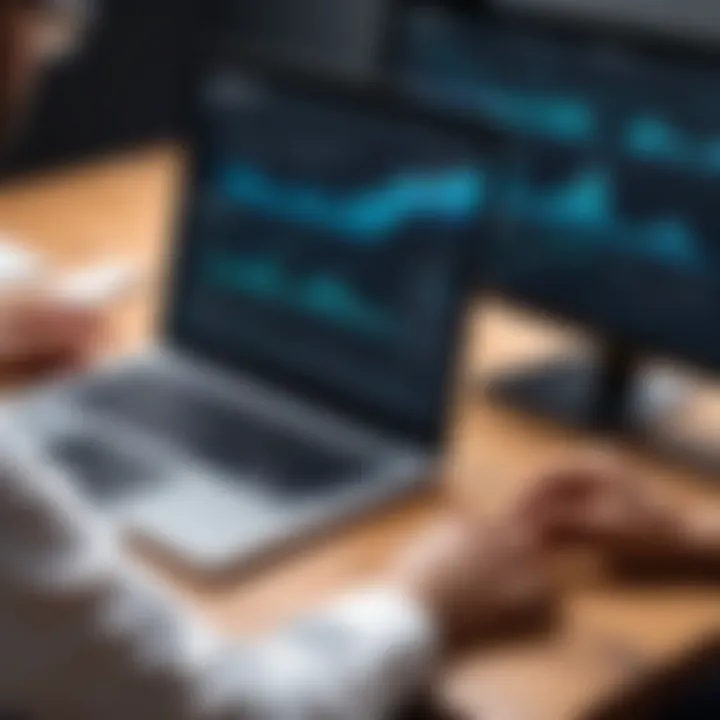
Such strategies enhance collaboration between marketing and sales teams. Data-driven decisions become the norm, promoting a culture of accountability and performance tracking. Lead scoring is not just a system to rank leads; it is a powerful tool for resource management that can lead to overall growth.
Managing Challenges in Lead Scoring
When employing an infer lead scoring model, it is crucial to recognize the potential pitfalls that can undermine its effectiveness. Managing challenges in lead scoring is central to ensuring that the system works efficiently and delivers accurate, actionable insights. This aspect not only influences the immediate sales efforts but also impacts long-term strategic planning. By identifying common issues and developing strategies to address them, organizations can improve the overall quality of their lead management processes.
Common Pitfalls
Several common pitfalls can hinder the success of lead scoring systems. Awareness of these issues is the first step toward mitigation:
- Over-reliance on Historical Data: Exclusive dependence on past data can skew predictions, especially in rapidly evolving markets. Consumer behavior can shift, and what worked yesterday may not hold today.
- Neglecting Behavioral Signals: Focusing only on demographic information often leads to misjudgment. Engaging with leads and observing their behavior online can provide critical insights not captured through traditional metrics.
- Inconsistent Scoring Methodologies: Applying different criteria or changing the scoring model without proper communication can confuse teams and lead to inefficiency. Consistency in scoring is key to ensuring reliable results.
- Ignoring Feedback Loops: Failure to incorporate feedback from sales teams can result in a disconnection between leads that are assigned high scores and those leads' actual performance in the market.
Addressing Inaccuracies
Inaccuracies in lead scoring can misguide marketing and sales efforts. Addressing these inaccuracies demands a multi-faceted approach:
- Regular Model Re-evaluation: Lead scoring models should be revisited at regular intervals to ensure they still meet the changing dynamics of the market. Every quarter could be a potential check-in point.
- Incorporating Multi-Source Data: Utilizing both internal and external data sources can help enhance accuracy. Cross-referencing traditional metrics with behavioral data helps build a more nuanced understanding of a lead's potential.
- A/B Testing: Continuously run A/B tests on different scoring criteria. This enables teams to see which factors resonate most effectively with target audiences, allowing for refining of the scoring model.
- Utilizing Advanced Analytics Tools: There are several analytical tools available that can aid in pinpointing inaccuracies by providing data-driven insights. Tools must be integrated into the lead scoring system to enhance forecasting capabilities.
"A well-managed lead scoring system can transform insights into actionable strategies, thereby enhancing the conversion path from lead to customer."
Successfully managing the challenges of lead scoring is crucial for driving efficiencies and improving performance metrics. Failing to address these issues can lead to wasted resources and missed opportunities. Hence, investing time and effort into refining lead scoring practices can yield significant advantages in the competitive landscape of B2B marketing.
Technological Advances in Lead Scoring
In todayβs data-driven landscape, technological advances have become crucial for optimizing lead scoring processes. Businesses strive to harness technology to enhance accuracy and efficiency in identifying high-quality leads. The integration of sophisticated tools not only elevates the performance of marketing strategies but also aligns sales efforts with lead potentials. This section will elucidate how these advances impact lead scoring models, focusing particularly on AI, machine learning, and predictive analytics.
AI and Machine Learning Applications
Artificial intelligence and machine learning are redefining the parameters of lead scoring. Algorithms that evolve and adapt to new data sets can outperform traditional models. Businesses employ these technologies to analyze vast quantities of historical data, identifying patterns and behaviours that humans may overlook. This ability to process multiple data points ensures better lead scoring accuracy.
Some significant benefits of using AI and machine learning include:
- Improved Predictive Capabilities: AI systems can project future behaviors by analyzing past interactions.
- Adaptive Learning: These systems refine their models based on real-time data, allowing for continuous improvement.
- Scalability: Technology can handle growing amounts of data without a proportional increase in resources.
Maintaining these systems, however, requires significant expertise and resources. Businesses must invest in training personnel and managing the implementation of such technology to reap the benefits.
Predictive Analytics Utilization
Predictive analytics represents another vital technological advancement in lead scoring. Companies can forecast outcomes through statistical algorithms and machine learning techniques. This enables them to determine which leads are more likely to convert based on historical data. By evaluating attributes such as engagement history and demographic information, organizations can prioritize their sales efforts more effectively.
Key elements to consider when using predictive analytics include:
- Data Quality: Predictive models rely heavily on accurate and relevant data. Poor data quality can lead to misleading predictions.
- Integration Capabilities: Existing systems and tools must seamlessly align with predictive models for effectiveness.
- Ongoing Monitoring: Predictive analytics must be continuously monitored and adjusted as market conditions and consumer behaviors change.
The integration of predictive analytics into lead scoring is not merely advantageous; it is essential for organizations aiming to maintain a competitive edge. As the market continually evolves, these technologies will shape the future of lead management.
"Harnessing AI and predictive analytics allows businesses to transition from reactive lead scoring to a proactive, data-driven approach."
In summary, technological advancements have a pivotal role in transforming lead scoring. By leveraging AI, machine learning, and predictive analytics, businesses can enhance their lead management processes significantly. As the landscape continues to transform, organizations must adapt and integrate these technologies for optimal outcomes.
Future of Lead Scoring
The landscape of B2B lead scoring is evolving at a rapid pace due to advancements in technology and changing market dynamics. The future of lead scoring is critical not only for improving marketing ROI but also for strategic planning and long-term growth. Businesses must adapt to these changes to remain competitive. Assessing emerging trends and understanding their long-term implications will enhance the efficiency and effectiveness of lead scoring systems.
Emerging Trends
As organizations continue to invest in lead scoring technologies, several key trends have emerged:
- Integration of AI and Machine Learning: AI tools are increasingly being used to refine lead scoring models. They analyze patterns at scale, offering insights that may not be immediately visible through manual methods. This enables continuous improvement of scoring accuracy as data evolves.
- Real-Time Data Utilization: With the growing importance of immediate decision-making, companies are adopting technologies that provide real-time data analytics. This allows for timely evaluations of lead quality, enhancing responsiveness to market changes.
- Advanced Predictive Analytics: Firms can leverage predictive analytics to not only assess lead quality based on historical data but also anticipate future behaviors and trends. This foresight can be crucial for resource allocation and strategy adjustments.
- Emphasis on Customer Intent: Modern lead scoring models focus increasingly on understanding customer intent. By analyzing online behavior and engagement signals, businesses can better determine which leads are more likely to convert, shifting away from traditional methods that relied mainly on demographic information.
These trends indicate a significant shift towards a more sophisticated use of data and technology in lead scoring. Companies that embrace these changes will likely see substantial benefits in lead qualification and conversion rates.
Long-term Strategic Implications
Leaning into these emerging trends carries various long-term strategic implications:
- Sustained Competitive Advantage: Companies that effectively adopt advanced lead scoring practices can develop a competitive edge. By improving the accuracy and responsiveness of lead evaluations, organizations can outpace competitors who rely on outdated methods.
- Resource Optimization: With better lead scoring, firms can allocate their marketing and sales resources more efficiently. This ensures teams focus on leads that matter, reducing wasted effort and increasing overall conversion rates.
- Enhanced Customer Relationships: A deeper understanding of customer behavior leads to more personalized marketing efforts. Businesses that leverage technology can tailor their approach based on intent signals, improving customer satisfaction and loyalty over time.
- Data-Driven Decision Making: Organizations will increasingly rely on data to guide decisions. This cultural shift towards data-driven choices will help in evaluating lead scoring methodologies and will keep strategies aligned with consumer behavior and market trends.
"Adopting innovative lead scoring techniques is not just about keeping up; it's about leading the market and setting the standard for the future."